Imagine you're the leader of a nonprofit organization dedicated to enhancing the well-being of members of your community. To assess the impact of your programs on stakeholders' experience, you seek out and find a “Stakeholder Satisfaction Survey (SSS)", a measurement tool obtained from an industry colleague to gauge client satisfaction with programs.
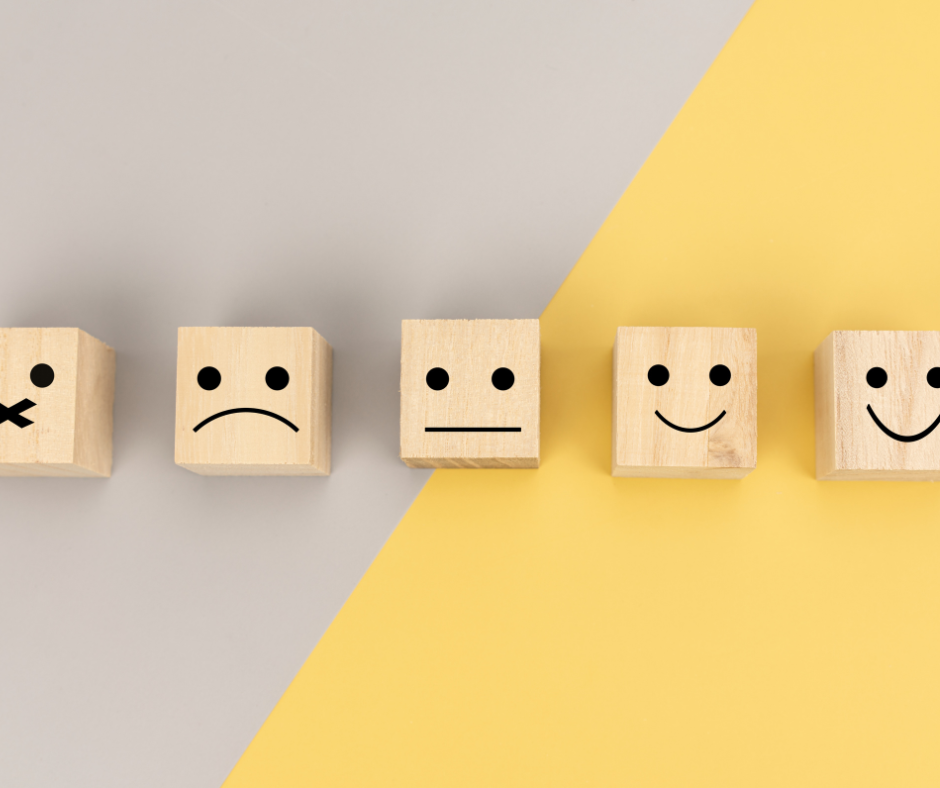
You diligently administer the SSS questionnaire to stakeholders and collect their responses. The SSS consistently shows high satisfaction scores, suggesting that stakeholders are pleased with the organization's efforts. Your data is consistent, and you are excited to share the results.
As you prepare to present these findings to board members and potential donors, however, you begin to wonder: "How is it possible that SSS consistently reports high satisfaction, yet the challenges to our community outreach and engagement efforts have remain unchanged?”
Could it be that your SSS is not capturing the full depth of stakeholder experience and perception? While the SSS provides consistent results, it may not be valid. The SSS is likely failing to capture the true essence of stakeholder experience as intended.
Unfortunately, this scenario plays out all too often. In an age awash with the need to back decisions with data, there is a tendency to use or create convenient survey measurement tools. Doing so, however, has the potential to greatly impact the quality of the data which in turn impacts the quality of the decisions subsequently made.
With Data Creation Comes Data Quality Responsibility
Understanding the concepts of reliability and validity is crucial when collecting survey data, as flawed measurement can result in incorrect conclusions. This becomes especially significant when the outcomes hold relatively high stakes, such as impacting resource allocation. Therefore, it is essential to ensure the collection of accurate data.
This distinction emphasizes the existence of both high- and low-quality survey measurements. High quality surveys yield dependable data and essential insights, offering valuable glimpses into the heart of concepts being investigated.
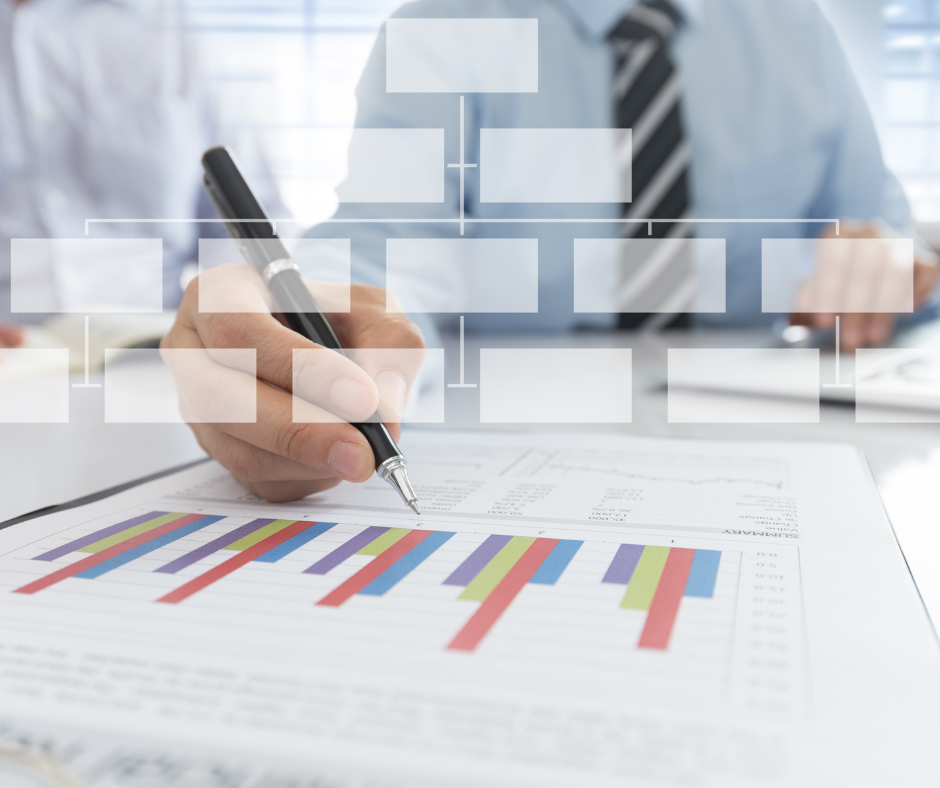
Confidence in Results: Ensuring validity and reliability means stakeholders can have confidence in the survey's findings.
Decision Making: Accurate and consistent data leads to informed decision-making in organizations.
Resource Allocation: Time, effort, and money can be better allocated when you're confident about your data's quality.
Ensuring Objectivity: A valid and reliable survey is less susceptible to biases, providing a clearer, more objective view of the scenario.
Conversely, low quality surveys generate unreliable data, ultimately leading to misguided conclusions and actions.
To trust the data, we must be able to ensure the reliability and validity of the measurement tools we are using to collect it. All too often, we inherently trust the measurement tools and data we acquire, assuming somebody else has established reliability and validity.
We must ensure that the response to the question: "Is the data generated by this survey reliable and valid for utilization?", is an unequivocal, “YES!”
In his book, People and Data (2023), Tom Redman makes the point that, in business, we are now all either consumers of or creators of data in our daily occupations. Data is an organizational asset that impacts decision-making so the responsibility of data quality becomes everyone’s responsibility.
Being data savvy isn’t just for the tech suite, it helps to ensure quality in the data supply chain. So, before grabbing the next “SSS” floating around, empower yourself with some data savvy to understand and test the reliability and validity of the survey measurement BEFORE you collect the data.
Understanding the World Around Us
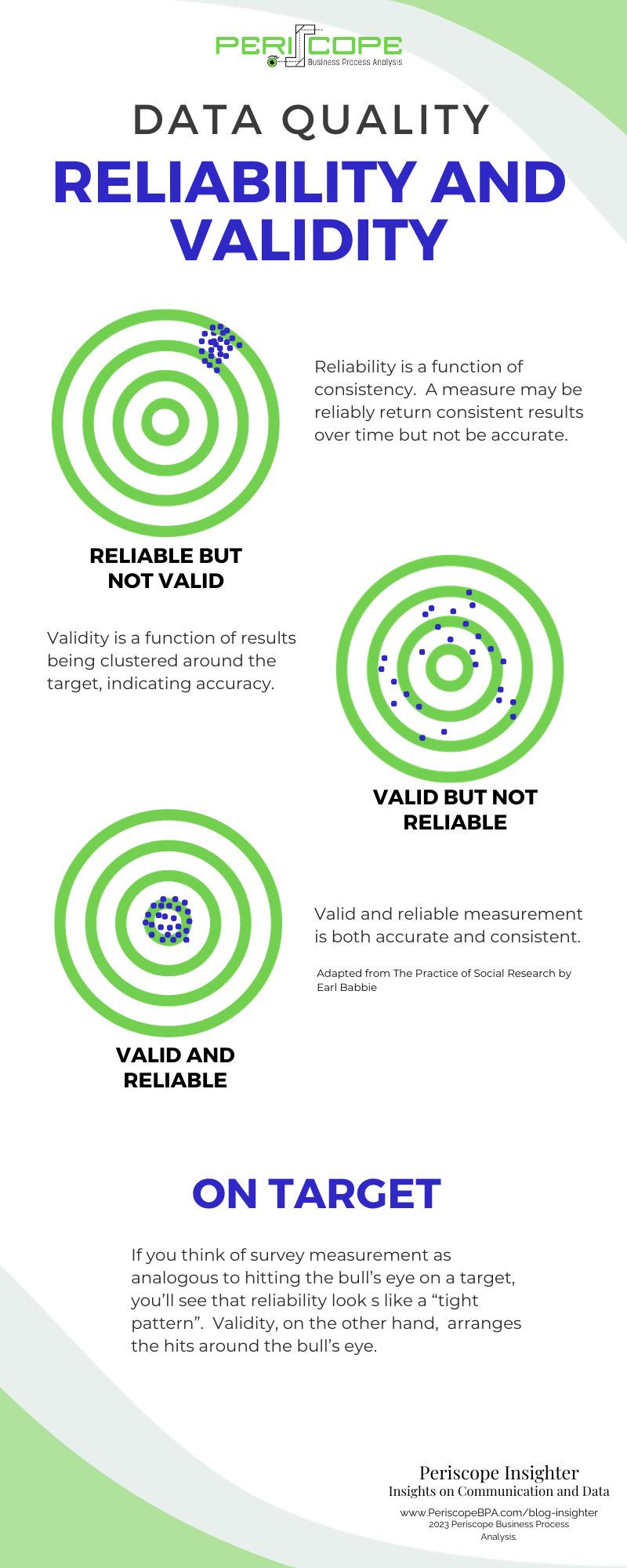
At this point, you may be asking yourself whether a construct like “satisfaction” can even be measured. While there is no perfect nor ideal way to measure sociological constructs, with the help of science we can effectively conceptualize concepts for investigation.
In the book, The Practice of Social Research (Babbie, 1995), the point is made that concepts are simply mental images that don’t actually exist in the real world, so they cannot be measured directly. “Stakeholder Satisfaction” is such a concept. However, we can bring together observations and experiences that have something in common. So, while it is not possible to measure the concept directly, we can measure the things that our concepts summarize.
The conundrum exists that most of the concepts we want to investigate have many nuances, yet distillation down to a survey requires specificity. For example, volunteer satisfaction is more than “the ability of getting help when needed”, a survey item found on the reliable and valid volunteer satisfaction measurement, the Volunteer Satisfaction Index (VSI).
The only justification for giving any concept a particular meaning is utility. -Earl Babbie
Ultimately, while there are limitations to any operationalized construct, we can accept them if we strengthen the likelihood that we are indeed capturing the true idea by establishing both reliability and validity.
Assessing Data Reliability and Validity in Survey Measurement
Survey design encompasses numerous elements that impact the data's quality, including factors such as the time and effort required by respondents, question sequencing, the scale's point count, and the arrangement of question-answer options. But central to all of this is reliability and validity.
For example, if you are designing a volunteer satisfaction survey and you consider asking the question “The convenience of this job to my schedule”, are you asking the right question? In other words, to what extent is this question measuring the concept of volunteer satisfaction? And how well will this question elicit the same kind of results? The first question considers validity, and the second question the reliability.
So, how do you know? The following methods assess the reliability and validity of survey measurement data.
Reliability: Consistency Across Time and Context
Reliability is the repeatability or consistency of a measure. If we were to repeat the survey, would we get the same or very similar results?
In an ideal world, if we ask a survey question of a participant, we should get the same answer irrespective of the interviewer asking the question or the day of the week.
Establishing Survey Measurement Data Reliability
Test-Retest Reliability This assesses the consistency of results when the same survey is administered at two different points in time.
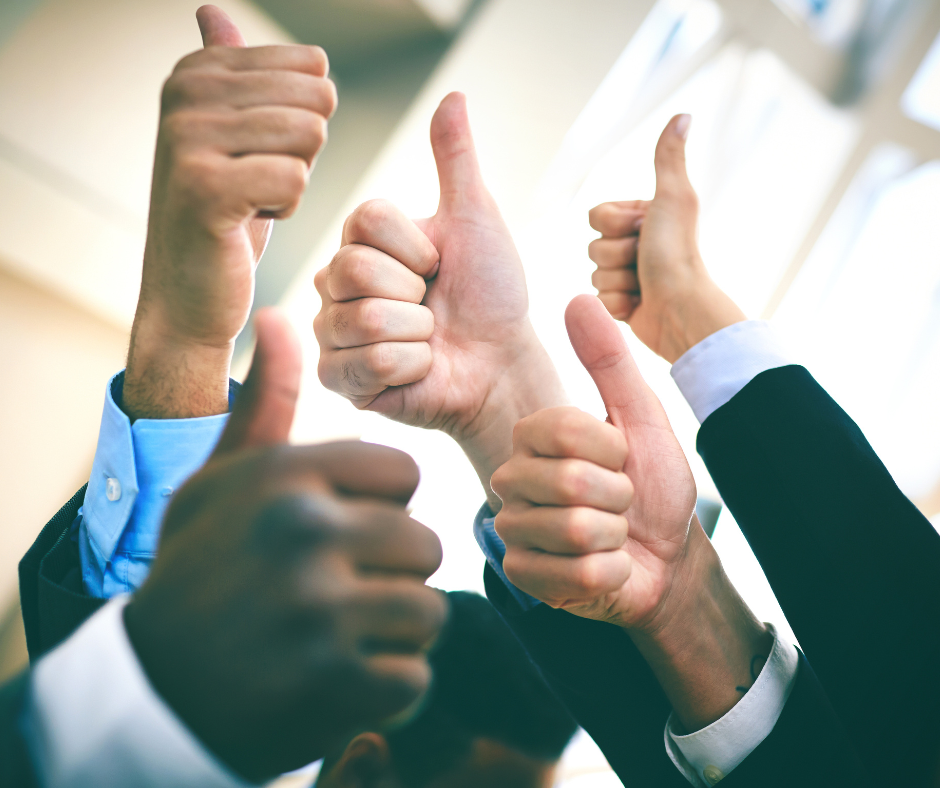
Inter-Interviewer Reliability This examines the consistency of results when the survey is administered by different interviewers.
Internal Consistency Often measured using Cronbach’s Alpha*, it assesses how closely related a set of items are as a group. For example, if a survey has multiple questions about a respondent's attitude towards participation efficacy, are the answers consistent across those questions?
Split-Half Reliability This involves dividing a survey into two halves and comparing the results. The consistency between these halves indicates reliability.
Validity: Measuring What We Intend to Measure
Validity is all about the accuracy of measurement. In essence, does our tool or survey measure what it's supposed to measure? There are several ways to establish validity in survey design.
Establishing Survey Measurement Data Validity
Content Validity: Does the survey content appropriately cover the concept it’s supposed to? For instance, a questionnaire on volunteer satisfaction should cover a range of topics from organizational support and belonging to the impact of their participation.
Criterion Validity This looks at how predictive a measure is. If a survey predicts a certain outcome, does that outcome actually happen? For instance, if a survey predicts intent to remain based on level of engagement, is it accurate?
Construct Validity This assesses how a tool measures a theoretical construct or trait. If a survey claims to measure "leadership qualities", does it touch upon facets like communication, empathy, and decision-making?
Face Validity This is the most basic level of validity and refers to whether a survey or tool superficially appears to measure what it's supposed to measure. It's based on initial perceptions and judgments. For example, if a test looks like it's measuring intelligence, then it has face validity.
Reliability vs Validity
RELIABILITY | VALIDITY | |
What is it telling you? | The extent to which the results can be reproduced when the research is repeated under the same conditions. | The extent to which the results really measure what they are supposed to measure. |
How is it assessed? | By checking the consistency of results across time, across different observers, and across parts of the test itself. | By checking how well the results correspond to established theories and other measures of the same concept.
|
How do they relate? | A reliable measurement is not always valid: the results might be reproducible, but they’re not necessarily correct. | A valid measurement is generally reliable: if a test produces accurate results, they should be reproducible. |
Going back to our Stakeholder Satisfaction Survey (SSS) scenario at the beginning of this article, the adopted measurement provided consistent responses each time asked, but did not have validity. Likewise, a valid measure that is measuring what it is supposed to measure does not necessarily produce consistent responses if the question can be interpreted differently by different respondents each time asked. A measurement survey used for data collection must have both reliability and validity.
In Conclusion
When delving into the intricate realm of survey design and data collection, two fundamental pillars that bolster its utility are reliability and validity. These are foundational elements that ensure the data collected is not only consistent but also paints an accurate representation of the situation or attribute being examined.
Incorporating and understanding reliability and validity in survey design is not just a theoretical exercise but a practical necessity and responsibility of being a data creator. In an age awash with data, ensuring the quality of the data we rely on sets the stage for genuine insights and impactful actions.
References
Babbie, E. (1995). The practice of social research (7th ed.). Wadsworth Publishing Company.
Galindo-Kuhn, R. & Guzley, R. (2001). The volunteer satisfaction index: construct definition, measurement, development, and validation. Journal of Social Service Research, 28 (4), 45-68.
Redman, T. C. (2023). People and data. Kogan Page.
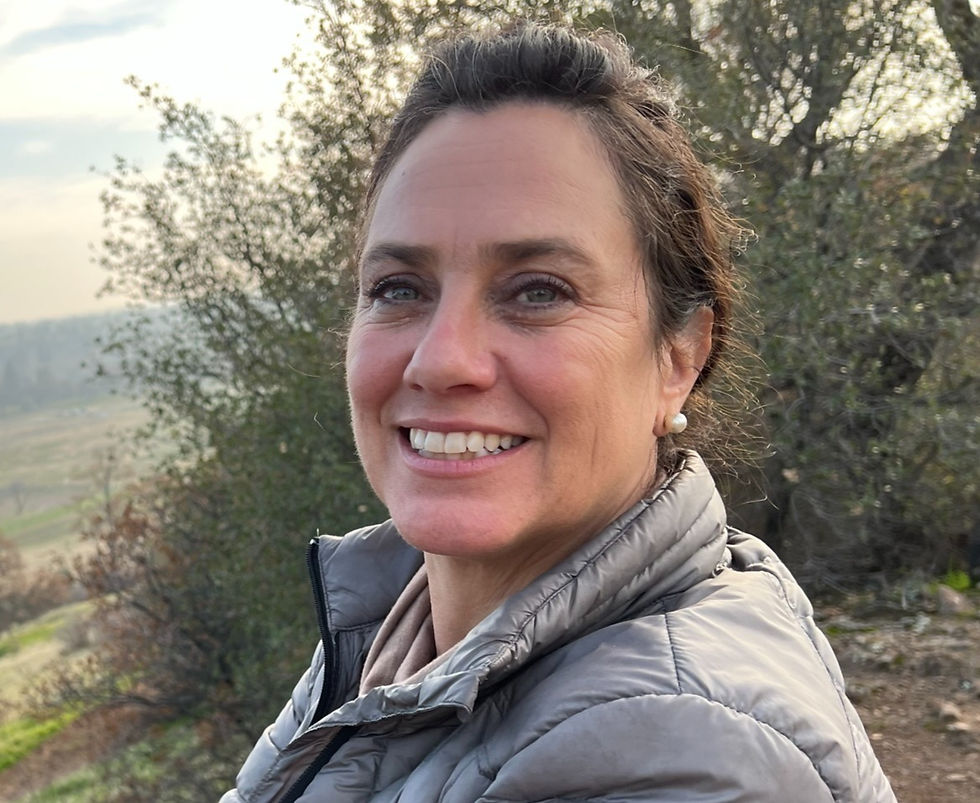
Roseanna Galindo is Principal at Periscope Business Process Analysis and a champion for data literacy, the human experience in healthcare, and leaders of volunteers everywhere. Learn more about Roseanna and her blog, The Periscope Insighter, by reading the opening post, Venn The Time Is Right
Roseanna is available for training, keynotes, and executive coaching.
Visit PeriscopeBPA.com for more information or click on the button below to schedule a time to talk
If you have found this article insightful, please share on social to help other like-minded business leaders to find their way here.
Comment. Share. Like. Subscribe. Thank you!
Comments